BACUDA:
Bringing Innovations to Customs Data Analytics
We studied the human-in-the-loop inspection scenario, where an AI-assisted algorithm supports officers by recommending a set of imported goods to be inspected.1,2,3,4 In the customs domain, officers determine whether the item is fraud and correct the amount of imposed duties.
In [1], we proposed an explainable tree-enhanced model to detect frauds and maximize revenues. However, a fraud detection system focused on high-risk items may face unexpected performance drops due to concept drifts. With add-on exploration strategies proposed in [2], the system will be maintained much more stable and robust against concept drifts. The amount of exploration needed for the system can be adaptively determined through the performance analysis of the current model and by measuring how much the underlying distribution changes [3]. Lastly, we conceptualized a knowledge-sharing platform for data-hungry regions to build their detection system with minimal support [4] and built a synthetic import declaration dataset to publicize the use of data and models [5].
For a glance at our current work, have a look at our papers:
[1] DATE: Dual Attentive Tree-aware Embedding for Customs Fraud Detection (KDD 2020): [Link] [Talk]
[2] Active Learning for Human-in-the-loop Customs Inspection (IEEE TKDE): [Link] [Github]
[3] Customs Fraud Detection in the Presence of Concept Drift (IncrLearn workshop at ICDM 2021): [Link] [Talk]
[4] Knowledge Sharing via Domain Adaptation in Customs Fraud Detection (AAAI 2022): [Link]
[5] Customs Import Declaration Datasets: [Link]
Snapshot from our weekly meeting:
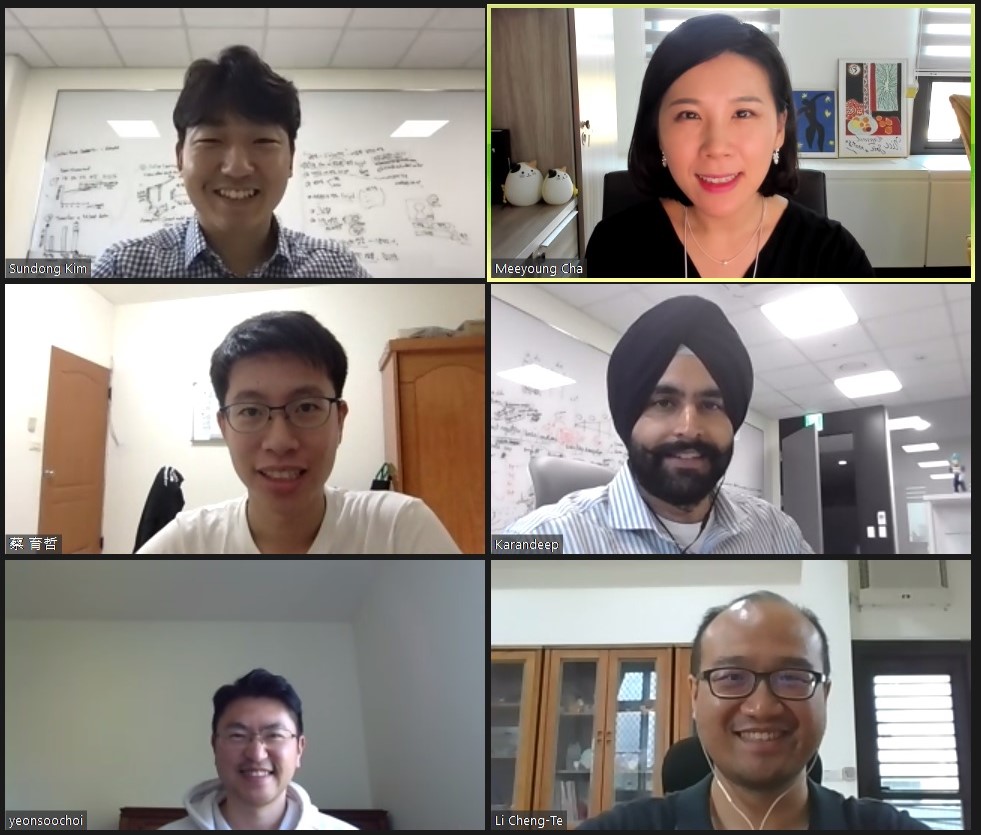
If you are interested in this project, feel free to contact Dr. Sundong Kim (sundong@ibs.re.kr), a research fellow at IBS Data Science Group.
- http://www.wcoomd.org/en/media/newsroom/2020/may/wco-bacuda-experts-develop-and-share-a-neural-network-model.aspx
- http://dongascience.donga.com/news/view/37042
- https://www.yna.co.kr/view/AKR20200529072100063?input=1195m
- http://www.wcoomd.org/en/media/newsroom/2020/march/nigeria-customs-embraces-data-analytics-with-the-wcos-support.aspx