How to interpret multiple topics from online reviews?
The abundance of online review data includes a broad spectrum of subjects, including web platforms and mobile apps, as well as offline stores and businesses, products and contents, and even humans. The wealth of review data has become the source of a number of recommender services that help people collect information quickly and make decisions. Comprehending the recurrent common topics underscores the shared concerns that emerge from diverse perspectives.
“How can you understand the interrelationships among topics?”
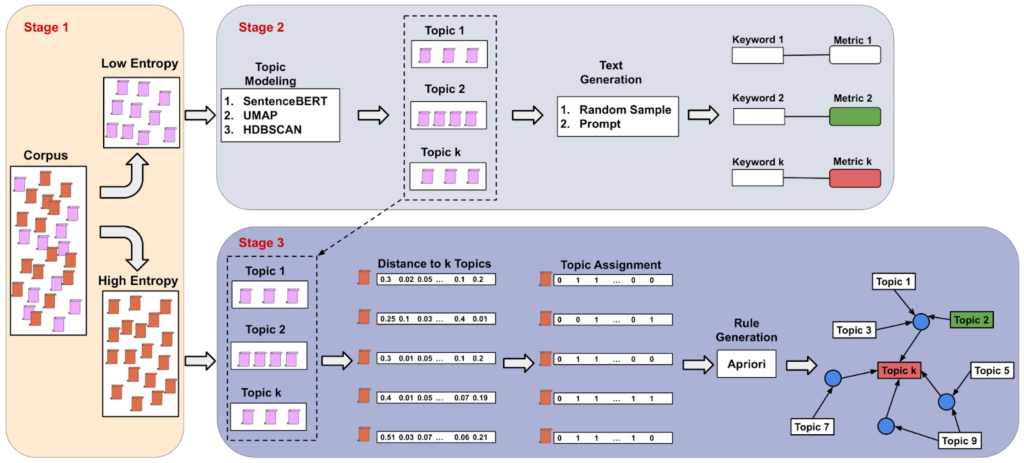
We propose a new multi-topic modeling and reasoning method that uses information theory, generative models, and association rules to identify and interpret topics with high-level labels. The method consists of three stages: partitioning the corpus into low- and high-entropy texts, finding focused topics and metrics in low-entropy texts, and assigning multiple topics and stimulating logic rules in high-entropy texts.
Here are significant topics identified from low-entropy data, with five random samples used for generating topic labels, together with average ratings.
1. Job Description
2. Issues and Criticisms
3. Workplace Satisfaction
4. Challenges
5. Work Schedule
6. Company Management
7. Work-life Balance
8. Team Dynamics
9. Company Culture
10. Job Insecurity
11. Learning and Growth
12. Office Politics
13. Compensation
14. Contractor Treatment
Relationships between Topics
After assigning multiple topics to high-entropy reviews, we stimulate logical rules among topics, augmenting the interpretation and unfolding intertwined relationships. The first six rules for topics “job insecurity” and “contractor treatment” as rule consequences are visualized below, where these two topics are least satisfactory among all identified topics, and the most positive topic, “learning and growth,” is largely driving them.
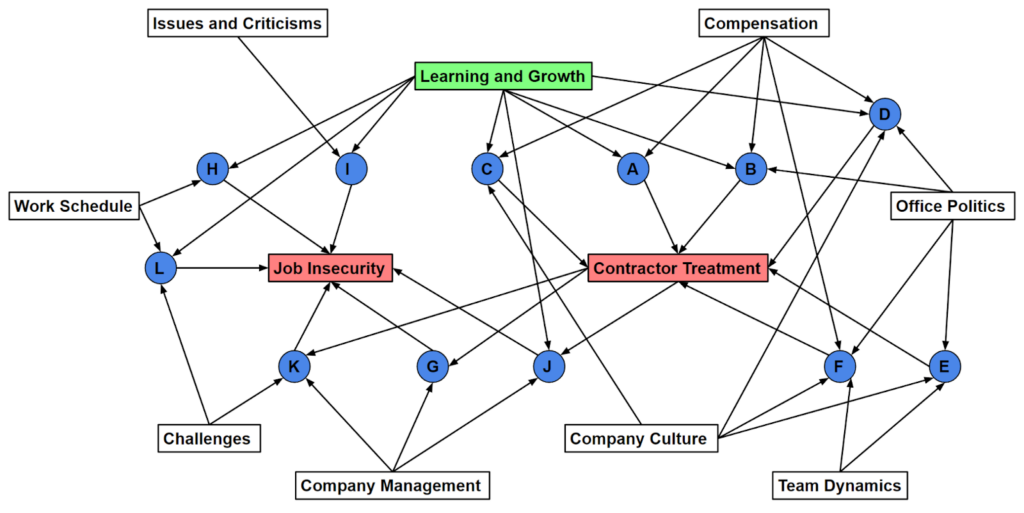
The topic colored green represents the most positive attitudes, while red topics denote the most negative two perspectives.
This multi-topic modeling and reasoning method has been recognized as helpful and inspiring even across other research domains. Below is feedback from other researchers:
Chatbot researcher: “I’m amazed by the versatility of this framework—it’s incredible how well it can adapt to various fields! In my research area, it provides me with valuable insights into the emotional states of chatbot users and helps me understand the reasons behind their feelings based on the topics they discuss. What sets this framework apart is its ability to combine multiple topics and offer interpretations—a feature that is hard to come by in other frameworks.”
Climate researcher: “The idea of applying rule reasoning over multi-topic modeling results is novel, which stimulates underlying casual relationships among multi-labels. In climate research, we deal with multi-label data as well, and this research intrigues me to consider to embed rule mining approaches into my own future work.”
Medical image researcher: “The usage of generative AI for generating labels for textual data, followed by a thorough investigation, might also be applied in medical imaging research.”
Please feel free to reach out to Wenchao Dong (wenchao.dong@kaist.ac.kr) if you have any questions about this project.